Q&A: Everything You Need to Know About AI for Business Growth
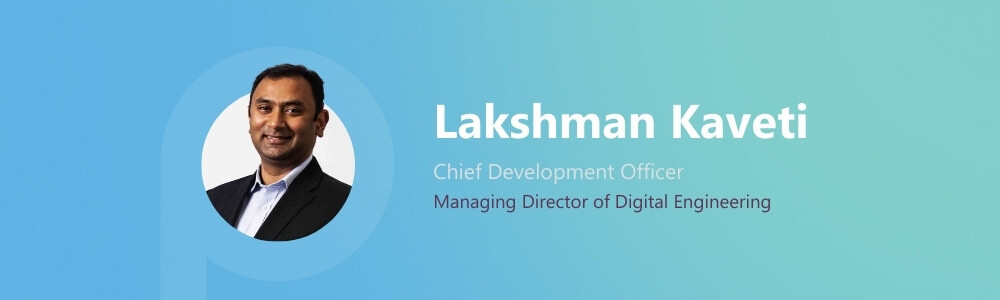
97% of business owners believe that AI will benefit their businesses, according to Forbes. There's huge potential for AI in business but how to make an AI vision a reality is where many businesses get stuck.
We recently talked with Lakshman Kaveti, AI Lead and Managing Director of Digital Engineering at ProArch, to hear his perspective on how businesses can leverage AI and be successful.
Key Takeaways
Where should organizations start their AI journey?
Before you dive in headfirst, make sure the whole team is on the same page about AI’s true potential. As Lakshman states, “I think there are different levels of knowledge one needs to build. What LLMs can do should go all the way to the board level. Everyone needs to have that understanding right that naturally evolves into finding the right use case to start with.”
Approach AI as the next step in your organization’s digital transformation. Starting there and having a collective understanding across the organization will help develop a path forward for integrating AI into your business.
How should organizations qualify and prioritize AI use cases?
Picking the perfect AI use case isn’t always straightforward, but the non-negotiable criteria to keep in mind when making the selection is you must have accurate and quality data. As Kaveti puts it, “If your data is messy and disorganized, any AI solution will be useless—an expensive gadget that doesn’t actually work.”
You need clean, accurate, high-quality data before you’re ready for AI. If you lack that, his advice is blunt: “Don’t embark on an AI journey at all. Focus on your data strategy first.”
To prioritize and qualify AI use cases, organizations should focus on high-ROI (return on investment) opportunities that align with business goals. Kaveti advises, “Wherever you are, your goal should be to get the most “bang per buck”. That’s where smart qualification comes.”
He recommends seeking external validation for proposed use cases, stating, “Don’t be afraid to bring in a consultant or AI consulting company to validate if this can actually be done.”
So, what makes an AI use case ‘the right one’? It solves a real problem delivering solid ROI and can be operationalized into existing workflows. Kaveti gives an example: “We integrated AI to clean data with human verification. That was the right use case because we had data that needed cleaning.” The ‘right’ use case provides tangible value by enhancing a process you already have.
How can organizations maximize their AI investment?
The range of investment on AI can be wide – a few thousand to hundreds of thousands. The key to avoid wasting time and money without positive return is to either fail fast or succeed fast.
Before investing, identify a specific use case and assess data availability. This will determine if the project is worthwhile. Leverage tools like Copilot or AI Studio to quickly validate feasibility.
“You don’t want to spend more than a few weeks validating. That’s how time and money is wasted,” Lakshman says. If you don’t have the capabilities in-house engage then you’ll want to engage with an AI consulting company, like ProArch, who has AI consulting services that can validate quickly.
Lakshman explained that you’ll want to work with a partner who has solution accelerators and matured frameworks that have been field-tested to work effectively so validation can be done in a matter of couple of weeks. Lakshman emphasizes that this rapid validation is crucial, as “time is money”.
What advice do you have for organizations that are hesitant about using AI?
Although AI might seem out of reach, it’s not. And the costs have come down. As Lakshman points out, “The costs are manageable for most use cases, barring a few highly data-intensive exceptions.”
Barriers like talent scarcity and technological limitations are no longer major hurdles. Pre-trained models and user-friendly platforms have democratized AI experimentation. “Ten years ago, we’d discuss building models from scratch. Now, existing models can likely handle most of what you need.”
The key is to start by identifying potential use cases rather than getting bogged down by the technology itself. His advice? “Go experiment. Figure out as many use cases as you can and find a way to validate them quickly and cost-effectively. That’s how you’ll know what’s going to work for your business.” AI is well within reach for businesses willing to experiment methodically.
What are responsible AI best practices?
Lakshman really emphasizes responsible AI practices. Start with a clear AI policy that lays out the rules for how employees use these powerful technologies responsibly.
A data protection program is critical too. You don’t want trade secrets or sensitive data being exposed by an AI model. Lakshman warns, “If you open it up too much, an AI could use something that’s protected or trademarked by someone else. I’d highly encourage using Microsoft Purview for data security. It solves data governance problems really easily.”
To avoid exposing sensitive data, you need robust safeguards with proper checks and balances and collaborating closely with security teams. “Talk to your CISO team about security policies. Their guidance ensures you don’t accidentally share or train models on data that shouldn’t be accessed.”
When you’re building and training AI models, especially generative ones, Lakshman advises following the HHH AI framework. Helpful, honest, and harmless. Part of responsible AI is mitigating hallucinations – instances where models generate false or misleading information due to issues like biased training data or overfitting. The consequences can range from spreading misinformation to reputational damage and safety risks in critical apps. Lakshman stresses strategies like using high-quality training data, structured data templates, refining prompts, and defaulting to human fact-checking to catch and correct hallucinations.
To wrap up here, what would be your suggestion for continuous maintenance of AI and what type of skills are needed?
Continuous maintenance of AI systems is crucial. AI models are not a one-and-done effort. AI models require retraining as user needs and data evolve. To handle this effectively, implement an LLM Ops or ML Ops framework. “The purpose of these frameworks is to holistically handle all aspects of the AI use case lifecycle including model selection, customization, retraining, productionizing, and maintenance.”
The key is having the right expertise on board to navigate this continuous improvement journey effectively. Having comprehensive monitoring and observability setups is absolutely critical. As Lakshman stated, “telemetry is going to be your best friend for keeping an eye on usage, performance, and any potential hiccups. You need that visibility into whether the AI is actually being utilized or not.”
Knowledge management is key too. Properly documenting processes, best practices, and enabling knowledge transfer helps ensure smooth sailing when team members change. Having an in-house data science expertise becomes invaluable here. AI is a multidisciplinary effort requiring data science, engineering, DevOps, and domain expertise.
At the end of the day, you have to continuously track the real-world value and impact the AI is delivering. That means setting up the right KPIs and metrics to measure desired outcomes. And be ready to adapt as user behaviors evolve over time. User behavior changes necessitate AI system adjustments, so regularly gather user feedback and analyze usage patterns to identify emerging trends requiring model updates.
What is the future for AI?
Right now, companies are mostly using AI in business to boost productivity for teams like finance, operations, marketing, and sales. Copilot for Microsoft 365 is a good example of that. But Lakshman predicts a shift is coming. Instead of just enhancing manual workflows, AI will start running on autopilot - autonomously executing tasks without human intervention.
As Lakshman puts it, “There will come a time where things will happen without humans needing to ask. Operationalizing AI is reaching peak productivity where things happen automatically,” Lakshman says.
For example, instead of manually triggering an AI to summarize text, the whole process could run autonomously - summarizing new content, seeking your approval, then sharing the summary.
The key, according to Lakshman? Building enough trust in the AI models first. "Automation kicks in once the confidence is there. Then we can make techniques smarter by adding our own prompts and contexts." So, while AI boosts productivity today, Lakshman sees a future where organizations let AI fully automate processes - once they develop that trust in the models.
With deep expertise in implementing AI technologies like generative AI and machine learning, ProArch’s AI consulting services deconstruct business problems and integrate AI where it makes the greatest impact. Reach out to us to work with an AI consulting company that turns obstacles into opportunities.

Assistant Manager Content Parijat excels at transforming complex technical ideas into clear, engaging stories that help businesses make smarter decisions. Her expertise shines in crafting impactful email campaigns, social media content, blogs, and more to drive demand generation and support sales. With experience in Oracle, Cloud, and Salesforce, she combines creativity and strategy to deliver meaningful results.